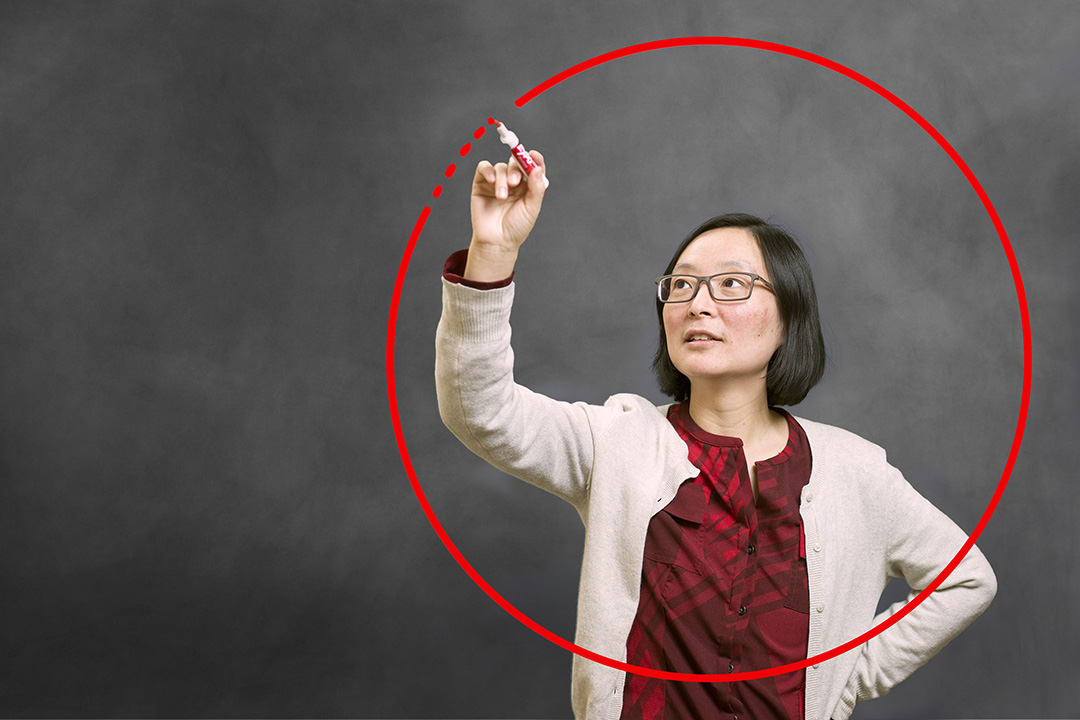
The data gap
All scientific research depends upon reliable data, but it can be difficult to obtain and is often incomplete or flawed. Juxin Liu uses statistical tools to help health science researchers account for and analyze imperfect data.
By Mari-Lou RowleyWe’ve all likely done it—skewed the truth when asked about our weight, exercise or drinking habits. When trying to obtain accurate information on public health risks, however, these fibs can adversely affect the outcome of a study. Case in point, smoking while pregnant is underreported on health surveys, despite the increased risks of premature delivery and low birthrates that can lead to infant mortality.
Statisticians understand the world in terms of data: information gained by measuring or observing variables. The smoking status of pregnant women, long-term nutrition intake and physical activity are examples of variables that are difficult or costly to observe. Other variables in health science research are impossible to observe, such as depression or quality of life.
“These are more complicated problems,” said Juxin Liu, professor in the Department of Mathematics and Statistics. “Conceptually you can define these things, but how do you quantify them?”
Unveiling the “truth” masked by imperfect data is a vital area of research for statisticians like Liu.
Breast cancer is the most common cancer in women worldwide, and although early screening programs have reduced deaths from the disease, proper diagnosis—particularly of hormone receptor (HR) status—is crucial to effective treatment. For example, post-surgery drugs that are highly effective in estrogen-receptor positive tumours are not nearly as effective in tumours that are estrogen-receptor negative.
But HR status is difficult to measure. It is also costly and depends on multiple factors, including specimen handling, tissue fixation, antibody type, staining and scoring systems—all of which are subject to error. Liu was lead author on a study of HR misclassification errors, working with her former PhD supervisor at the University of British Columbia and clinicians from the University of Chicago.
Until 2010, the guidelines used by clinicians called for a tumour to be diagnosed with a positive HR status if 10 per cent of the sampled cells tested positive. Since then, the cut-off has been reduced to one per cent. Intuitively, one would think this a good thing, because the change increases the test’s sensitivity—the chance of correctly identifying HR positives. However, reducing the cut-off also decreases the specificity, meaning there is a greater chance of falsely identifying negatives as positives. The Bayesian methodology proposed by Liu and her colleagues takes this “tug-of-war” relationship between sensitivity and specificity into account in adjusting for misclassification errors.
Bayesian tools use historical information, or prior knowledge, in addition to the empirical data being analyzed. In the breast cancer study, the professional knowledge of clinicians was combined with the cut-off change to create a more accurate statistical analysis. It is one of the ways statisticians such as Liu assist other researchers in analyzing data that are misclassified, unknown or incomplete.
“For this study, the prior information about sensitivity and specificity comes from the clinicians’ expertise,” said Liu.
It’s her job to fill in the gaps.