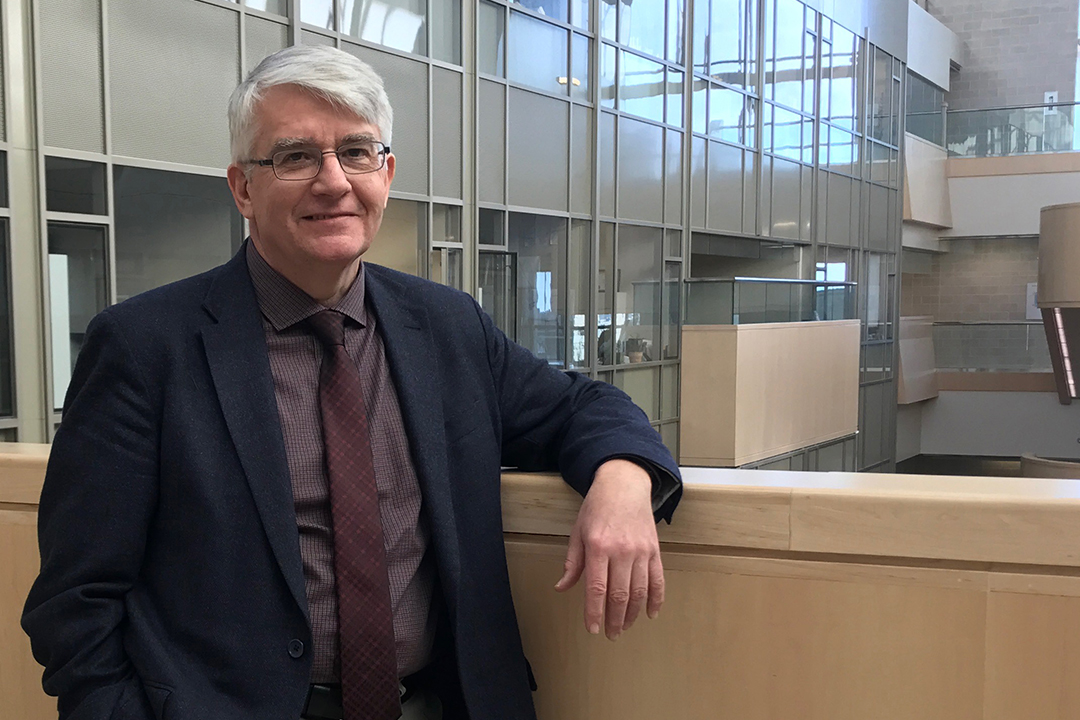
Creative campus collaboration
An ongoing collaborative research project between the College of Medicine and the Department of Computer Science at the University of Saskatchewan is focused on improving the accuracy of medical imaging diagnosis.
By Marg SheridanThe team is hoping to not only speed up how quickly thyroid nodules are diagnosed, but improve how accurate the diagnosis are.
“From the research side, we have strong potential to be able to reduce the overall system costs by avoiding unnecessary invasive procedures in some patients,” said Dr. Paul Babyn, the head of the department of medical imaging. “And that would obviously save that individual patient from having a biopsy or surgery when they don’t need it.”
The potential to improve the diagnosis process builds on Babyn’s previous work with Dr. Gary Groot (surgical oncology) and professor Ekta Walia (computer science) on thyroid tumours.
“We know that use of thyroid reporting systems, with the human doing the interpretation of the images, improves results,” Babyn explained. “Use of a scoring and reporting form provides information in a standardized fashion for both the surgeon and pathology and reduces variability.
“We know that there are limitations to the application of the human scoring system, as they require additional time and expertise not always available within a busy practice setting.”
The scoring system allows trained radiologists to classify thyroid lesions as either “not suspicious,” and therefore not in need of biopsy; “very suspicious,” and in need of an immediate biopsy; or “less suspicious,” but still in need of biopsy or follow-up. Getting it correct right off the bat can reduce the number of patients needlessly undergoing biopsies or surgical procedures.
“So there’s the potential for a machine learning system to provide a reproducible interpretation with the scoring system,” Babyn continued. “That would allow improved output for the radiologist, as well as improved clarity for the surgeons.”
Babyn had already been working with computer science researchers in the College of Arts and Science to review computer-assisted diagnosis for thyroid cancer. But with ongoing advances in deep- learning, they decided to apply some newer technology to the problem of thyroid nodule diagnosis.
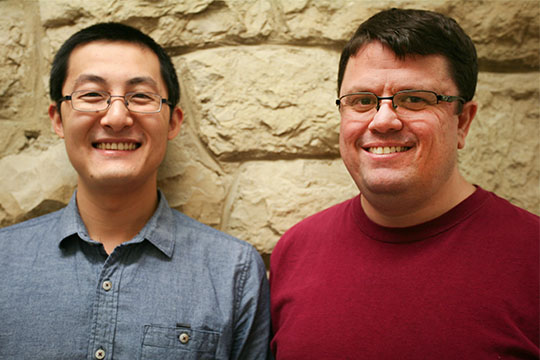
“We’re using techniques that are called deep-learning algorithms,” explained Mark Eramian, an associate professor in computer science who has been helping lead the research.
“We are analyzing the ultrasound images with the computer and trying to predict with high accuracy whether these are likely to be benign or whether we should do further, and more invasive, tests to be sure that they aren’t malignant.”
Traditional machine learning requires a person to determine the distinguishing features that a computer analyzes to make a determination—the kind of technology used by red-light cameras or with license plate recognition systems. But with deep learning, the distinguishing features are not deter- mined by a human. Instead machine algorithms are used to learn not only how to interpret the features to make the diagnosis, but also the features themselves.
“That allows many more possible distinguishing features to be considered than a human could reasonably consider in a lifetime,” Eramian continued. “We have a data set that is annotated so we know from the biopsies whether the nodules were malignant or benign, and learned features are attributed to those annotations. By considering and optimizing so many features, we end up with a better decision-making tool.”
Of the 2,500 images the team is using, 1,600 were selected as a training data set so that a deep neural network could learn the features and classifiers. Some 400 images, which the network had never seen before, were then used for testing so that it could make its prediction regarding the nodule, which the researchers could then compare to their results.
After having done 10 training/ validation/testing variations with the network, the team was getting an average accuracy of 92 per cent.
But Eramian explained that it’s not only the accuracy that’s important, but also the sensitivity.
“When you’re doing computer-assisted diagnosis of cancer, there are two ways to be wrong: you can say it’s malignant when it’s actually benign, or you can say it’s benign when it’s actually malignant,” Eramian explained. “The sensitivity is what percentage of the malignant cases were correctly labelled as malignant, and the specificity is what percentage of benign cases were correctly labelled as benign.
“Those two kinds of errors have very different costs: it is disastrous to conclude that a nodule is benign when it’s actually malignant, but the worst consequence of a benign module being labelled malignant is a biopsy that comes back negative. We can tune the classifier so that sensitivity is preferred over specificity. We might, for example obtain near-perfect sensitivity, successfully detecting malignant nodules, at the cost of labelling a small percentage of benign cases as malignant. Then we’re doing biopsies in those few benign cases, but probably still fewer than what we’re doing now.”
It’s not just a matter of Eramian’s team uploading images into the network, however. Since the images
have been sourced from hospitals and health systems from across the country, they arrive with varying imaging standards. Jianning Chi, a research assistant in computer science, has to manually match the scales (the pixels per centimetre) and image quality before they can be used. “We have to adjust the images to make sure they have the same scale, according to the network specifications,” Chi said. “I first detect the ticks (the measurement scale each ultrasound uses) on the images, and then resize the images so they have the same scale. I then locate the markers made by radiologists, which
show the regions of interest, before removing them from the image and filling in the gaps with approximations based on neighbouring textures, which ensures that the samples are noise-free.
“So I’ve been repurposing the samples to train the deep-learning network to improve its performance.”
The team expects to publish its research later this spring, which Babyn hopes will be followed by using the technology in clinical trials.
Marg Sheridan is an online communications co-ordinator in the College of Medicine.